One of the obstacles if you are trying to guide policy and protect people from COVID-19 is the bewildering amount of data available every day. We are awash in information, but everyone wants to distil insight that makes sense from this.
Policymakers need to make sense of the data so as to predict and manage what’s happening. To address this need, we developed a data-driven disease surveillance framework to track and predict country-level COVID-19 cases.
Read more: Contact Tracing Apps Can Work, If They Can Surmount Their Trust Problem
We are an international team of scientists working on novel ways of predicting diagnosis when presented with a serious infection. We start with model-based prediction of infectious diseases. Ultimately we are interested in improving treatment when the infectious agent is unknown. But for COVID-19, our methods are valuable for understanding and predicting the infection at the population level.
Our focus has been on infant infections in East Africa and in Southeast Asia. For over 15 years, we’ve worked with Ugandan hospitals, healthcare providers, planners, economists, engineers and policymakers on predictive mapping of infant infections and birth defects.
Read More: How to Make a Low-Cost Air Purifier to Reduce COVID-19 Transmission Indoors
Early in 2020 we began discussing ways to use our skills to contribute to the fight against the novel SARS‑CoV‑2, the virus causing COVID-19. We tapped into a wide network of experts, organisations and local authorities. They helped us adapt our approach to generate predictions for week-ahead COVID-19 case numbers.
We created a set of visualisation tools and graphs that are very easy to interpret. We worked closely with the Ugandan planners and economists to be sure that what we created together could be implemented. We built on a proven method that was already in use in the UK and several European countries, adapting it to the whole of the African continent.
Read More: Can Handwashing with Ash or Soil Prevent COVID-19?
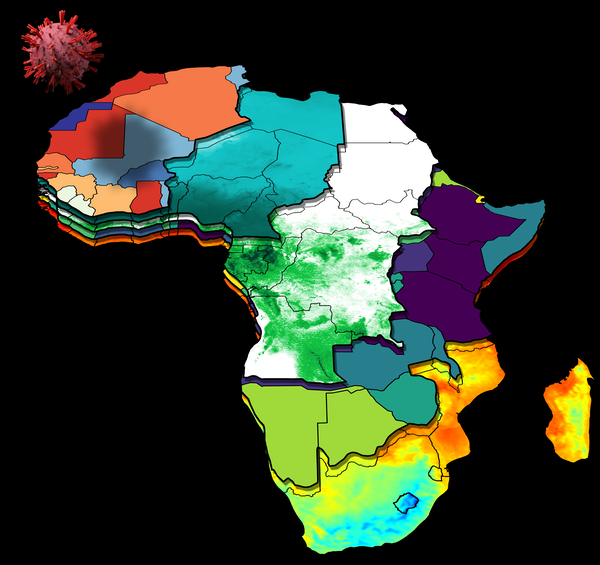
The model used in this study integrates multiple sets of data to predict COVID-19 transmission. Layers, from top to bottom: COVID-19 case counts, specific humidity, rainfall, population, and temperature. Photo: Andrew Geronimo
How the model is built
We used a relatively simple model, by mathematical standards, to base the computer codes. We incorporated the daily case reports from each country, as well as the characterisation of the Human Development Index for each country, population, the stringency of social measures to control the infection, and meteorological data. We took into account the cases in neighbouring countries, as well as whether the country is landlocked.
Our model used the case numbers reported up to and including the previous week, to predict the case numbers in the week ahead.
The model included past weather observations of temperature, rainfall, and specific humidity. Some of these environmental factors have been implicated in COVID-19 transmission outside of Africa. We modified the weather data for each country by adjusting for population. In Algeria, for example, where much of the population lives along the cooler, wetter coast, we emphasise or weight the weather data in proportion to where people live (and ignore weather data over unpopulated desert).
We took into account cross-border flow of goods and commerce, since for landlocked African countries, this is their lifeline for goods and services.
It is difficult to obtain population mobility data for Africa, so we used the cases in all other countries to test whether those cases were associated with the next week’s cases in nearby countries. This proved very effective.
We factored in the economics of different countries, as well as the evolution of real-time governmental stringency policies such as lockdowns and border closures.
And then we needed to see if we could get an understandable summary of the current state of the pandemic in Africa, and whether predictions a week or so ahead were accurate compared with the actual measured case numbers.
From predictions to policy
The predictions were much more accurate than we had anticipated. We employed a rigorous way of scoring predictions for each country. Only for a few countries were these predictions inaccurate (Burundi, Cameroon, Somalia, and Botswana). To visualise these predictions, we created a set of tools and graphs that are very easy to interpret. A policy person needs to see what the present situation is, how that current state got there from past events, and what will happen in the future. The websites we created will enable anyone to use a computer mouse to select their country, or the continent, and see the results of the model. No math or PhD required.
Read More: Eight Lessons Learned Designing a COVID-19 Response Ventilator
For the technical scientists in other countries or the global agencies, we are sharing our full code. We wrote this code in free open-source software, and all the data that the model uses is from free open-source websites, so that there are no barriers to implementation for anyone. Our methods stress data that is predictive, and automatically ignores data that is not contributing to an understanding of the cases next week. This is an old trick in economics, and we found that it works very well in predicting infectious cases.
The publication contains links to computer code that runs the model, and to the graphical display of these findings, for anyone to use.
Our colleagues in Uganda are finding this model useful in predicting cases a week ahead. They are using it to help inform social policies and lockdowns as they track the effect on cases, and plan for reopening as case pressure eases. Because the effect of control measures is delayed by a period of weeks, prediction enables better policy planning. But like so many things in nature, such as the weather, predictions beyond two weeks are often very uncertain.
Looking ahead
One of the clear implications from our findings is that pandemics are crises that no country can best manage on its own. We encourage neighbouring African countries to share data and cooperate on travel and border management.
This pandemic will end through massive vaccinations in Africa. Until billions of additional safe and effective SARS-CoV-2 vaccines become available for Africa, predictive methods such as this to help inform policy will be needed.
About the Authors
Steven J. Schiff is Brush Chair Professor of Engineering in the Departments of Neurosurgery, Engineering Science and Mechanics, and Physics at Penn State University in Pennsylvania (USA).
Andrew Geronimo is Assistant Research Professor in the Department of Neurosurgery at Penn State University.
Claudio Fronterre is Lecturer in Biostatistics at the Centre for Health Informatics, Computing, and Statistics at Lancaster University in Lancaster (UK).
Paddy Ssentongo is Assistant Research Professor at the Center for Neural Engineering in the Department of Engineering Science and Mechanics at Penn State University.
This article is republished from The Conversation under a Creative Commons license. Read the original article.